Authors: Haritha George & Simran Rana
Diffusion Tensor Imaging (DTI)
In 1992-93, Douek et al (1993) and Basser et al (1992) proposed a diffusion tensor to model the intrinsic diffusion properties of biological tissues. A diffusion tensor (DT) can be thought of as a 3x3 matrix that is symmetric and positive. Mathematically, it is an ellipse in 3 dimensional space, making it an ellipsoid. A symmetric equation, it has six unknown coefficients that need to be estimated.
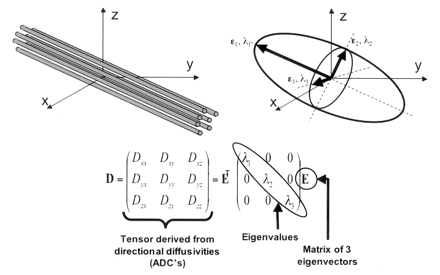
Left, fiber tracts having an arbitrary orientation with respect to scanner geometry (x, y, z axes) and impose directional dependence (anisotropy) on diffusion measurements. Right, the three-dimensional diffusivity modeled as an ellipsoid whose orientation is characterized by three eigenvectors (ϵ1, ϵ2, ϵ3) and whose shape is characterized three eigenvalues (λ1, λ2, λ3). (Adapted from Jellison, B. Field et al (2004))
Eigenvalue decomposition allows for the extraction of several rotationally invariant quantities such as the apparent diffusion coefficient (ADC), or the fractional anisotropy (FA), among others (Pierpaoli et al, 1996).
The FA map is certainly the most famous DTI anisotropy measure. It is a normalized measure between 0 and 1 that lights up the white matter. High FA values (0.7 and more) reflect voxels with highly organized fiber bundles in a single direction, whereas low FA values (0.15 and lower) reflect voxels with isotropic diffusion. Mid range FAs are harder to decipher which is why parallel and perpendicular diffusivities are used to complement FA to separate diffusivity along the principal and orthogonal directions of the tensor.
Advantages of DTI
One of the biggest advantages of DTI is its short acquisition time (5 min).
The diffusion tensor has powerful properties that one can exploit for diffusion MRI applications and clinical diagnosis. In fact, ADC, FA, parallel diffusivity, and perpendicular diffusivity maps are now a central part of several studies of neurological and pathological disorders like aging, Alzheimer’s disease, brain tumors, dyslexia, epilepsy, multiple sclerosis, schizophrenia, stroke, vascular dementia etc. (Jones, 2010).
DTI acquisition, reconstruction, scalar maps, and basic streamline tractography are now part of every manufactured MRI system. This opens DTI to a large number of neuroscience applications.
However, DTI is still not a “press button” procedure.
Limitations of DTI
Default parameters at the imaging console are not standard and are hard to select for the inexperienced user.
The most important limitation of the diffusion tensor model is its Gaussian diffusion model assumption. Hence, only a single-fiber population can be modeled at every imaging voxel. This is an important limitation when imaging voxels have multiple fiber populations crossing or highly curving fiber bundles. It is estimated that between 66% - 90% of white matter, voxels with FA above 0.1 have multiple fiber crossing configurations.
In the worst case, this means that the DTI assumption is violated more than 2/3 of the time. If one is aware of DTI limitations and is careful as to how results are interpreted and used, it remains a powerful tool. Therefore, it is important to go beyond DTI.
Higher order models and new reconstruction techniques are needed to be able to describe non-Gaussian diffusion signals. Of course, high-order models have more degrees of freedom and, therefore, more diffusion-weighted images will be required for the problem to be well defined and well posed. This has led to the development of HARDI techniques.
High Angular Resolution Diffusion Imaging (HARDI)
HARDI was originally developed to overcome the limitations of DTI and solve the crossing fiber problem to make tractography more robust and provide new anisotropy measures beyond the classical FA and diffusivities computed from DTI. To overcome the limitations of the single Gaussian assumption, two families of techniques were explored, model-free techniques and model-based techniques.
In the previous blog, we talked about using VoxelBox for Traumatic Brain Injury (TBI) patients. We spoke about Edlow et al’s (2020) work where they mapped the structural sub-cortical Ascending Arousal Network (AAN) connectome on a clinical MRI scanner and used Higher Angular Resolution Diffusion Weighted Imaging (HARDI) tractography to map axonal connectivity based on directional water diffusion.
So why did the authors use HARDI and not Diffusion Tensor Imaging (DTI)? Dr. Greg Brown’s tweet says, "We know 95% of the brain is crossing fibres. Drop DTI go HARDI ''. The following section compares the two techniques and shows why HARDI is the clear winner.
DTI vs HARDI
Acquisition Parameters
The difference in the acquisition of HARDI and DTI is in the directions and b value with HARDI (64-direction, b=3000 s/mm2) and DTI (30-direction, b=1000 s/mm2).
Fiber Tracking
What’s more, HARDI overcomes the limitations of DTI in crossing fibers and curved areas allowing for robust tractography.
HARDI fiber tracking success rate is 98% which is significantly higher than the 64-direction DTI (50%) as well as the 30-direction DTI rate of 42% (Berman, 2013).
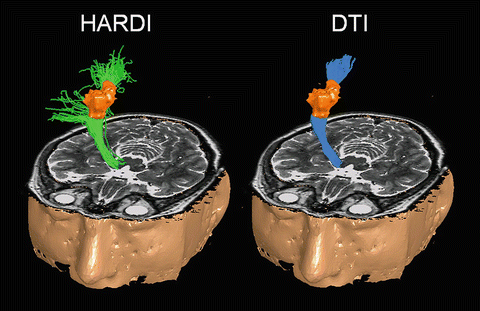
HARDI tractography of the motor system (green streamlines on left) is compared to DTI tractography (blue streamlines on right). The lesion is in orange. (Figure adapted from Berman 2009)
Tracking Cerebellar Connectivity
The cerebellum controls sensorimotor function, intellectual processing and emotional regulation. With the different types of cells regularly aligned in the cerebellar cortex, topographical relationships between the cells and folia are common across these regions.
Ubiquitous features of crossing axonal pathways within a folium, intersecting pathways from neighbouring folia as well as tangential fibers within the cerebellar cortex which were distinct from fibers linking the cerebellar cortex with the deep cerebellar nuclei and the brainstem make the tractography with DTI difficult. The ability to resolve axonal pathways from different neuronal elements within the cerebellar cortex as well as adult cerebellar neural circuitry and connectivity in both white and gray matter is possible with HARDI (Takahashi et al, 2013).
Brain Stem Tractography
Brain stem tumours are inoperable most of the time due to the presence of vital centres and tight packing of the fibres. As our advisor Dr Mazda mentioned, “Precise tractography of the brain stem, if possible, can become a very crucial factor in the decision making for surgical interventions”.
DTI fiber tracking fails to accurately follow white matter pathways through tract intersections. The midbrain serves important functions in motor movement, particularly movements of the eye, and in auditory and visual processing. Thus, using HARDI for auditory radiations can be a step closer to having a full brain stem tractography with HARDI in future.
Tumors
HARDI can have implications in tumor surgeries as well. Recent studies with HARDI + Compressed Sensing (CS) -based tractography displayed more compact fiber bundles compared with DTI. The most frequently used method for fiber tractography based on DTI is associated with restrictions in the resolution of crossing or kissing fibers and in the vicinity of tumor or edema. With CS techniques, HARDI acquisitions with a smaller number of directional measurements can be used, thus enabling the use of HARDI-based fiber tractography in clinical practice. Kuhnt’s (2013) study showed neuroanatomically plausible fiber bundles in the vicinity of tumor and peritumoral edema, which could not be traced on the basis of DTI.
Cortical Connectivity
Cortical connectivity is associated with cognitive and behavioral traits that are thought to vary between sexes. A novel HARDI tractography algorithm based on the Hough transform to extract tracts throughout the entire brain was used by Jahanshad et al in their 2011 study. HARDI detected cortical connectivity and interhemispheric connectivity (which was greater in women), in line with long-standing theories of hemispheric specialization. It is possible that differences in connectivity between sexes may be important to recognize in the Human Connectome.
Advances in HARDI over the years
Research shows that HARDI signals can be reconstructed model-independently using a spherical tomographic inversion called the Funk–Radon transform. The resulting imaging method, termed qball imaging (QBI), can resolve multiple intravoxel fiber orientations and does not require any assumptions on the diffusion process such as Gaussianity or multi-Gaussianity (Tuch, 2004). QBI has the advantage of being model-free and can reconstruct water diffusion orientation distribution function (ODF) from single-shell HARDI acquisition with relatively small b value (Li, 2014).
Constrained spherical deconvolution (CSD) is a new technique that, based on high-angular resolution diffusion imaging (HARDI) MR data, estimates the orientation of multiple intravoxel fiber populations within regions of complex white matter architecture, thereby overcoming the limitations of the widely used diffusion tensor imaging (DTI) technique (Jeurissen et al, 2011).
The noisy nature of diffusion-weighted (DW) images, however, affects the estimated orientations and the resulting fiber trajectories will be subject to uncertainty. The impact of noise can be large, especially for HARDI measurements, which employ relatively high b-values. The use of CSD over other popular HARDI methods such as Q‐ball imaging (QBI) is that, CSD is able to estimate multiple intravoxel fiber orientations more accurately than QBI.
The progress made in imaging techniques from DTI to HARDI is an exciting one and we at BrainSightAI want to explore the possibilities HARDI has to offer with our product VoxelBox. If you are interested in posing and answering questions related to neuroscience, please write to us at collaborations@brainsightai.com
________________________________________________________________________
References
Basser, P. J. (1992). Fiber orientation mapping in an anisotropic medium with NMR diffusion spectroscopy. In Book of Abstracts, in Society of Magnetic Resonance in Medicine (Vol. 1, p. 1221).
Berman, J. I. (2009). Diffusion MR Tractography As a Tool for Surgical Planning. Magnetic Resonance Imaging Clinics of North America, 17(2), 205-214.
Berman, J. I., Lanza, M. R., Blaskey, L., Edgar, J. C., & Roberts, T. P. (2013). High angular resolution diffusion imaging probabilistic tractography of the auditory radiation. American Journal of Neuroradiology, 34(8), 1573-1578.
Douek, P., Turner, R., Pekar, J., Patronas, N., & Le Bihan, D. (1991). MR color mapping of myelin fiber orientation. J Comput Assist Tomogr, 15(6), 923-929.
Brown, Greg, and Shauna Farquharson, n.d. https://twitter.com/gbsmrt/status/729199031017070593.
Jahanshad, N., Aganj, I., Lenglet, C., Joshi, A., Jin, Y., Barysheva, M., ... & Thompson, P. M. (2011, March). Sex differences in the human connectome: 4-Tesla high angular resolution diffusion imaging (HARDI) tractography in 234 young adult twins. In 2011 IEEE international symposium on biomedical imaging: From nano to macro (pp. 939-943). IEEE.
Jellison, B. Field, A. S., Medow, J., Lazar, M., Salamat M. S. and Alexander, A. L. (2004). Diffusion Tensor Imaging of Cerebral White Matter: A Pictorial Review of Physics, Fiber Tract Anatomy, and Tumor Imaging Patterns. American Journal of Neuroradiology, 25 (3) 356-369.
Jeurissen, B., Leemans, A., Jones, D. K., Tournier, J. D., & Sijbers, J. (2011). Probabilistic fiber tracking using the residual bootstrap with constrained spherical deconvolution. Human brain mapping, 32(3), 461-479.
Jones, D. K. (2010). Diffusion mri. Oxford University Press.
Kuhnt, D., Bauer, M. H., Egger, J., Richter, M., Kapur, T., Sommer, J., ... & Nimsky, C. (2013). Fiber tractography based on diffusion tensor imaging compared with high-angular-resolution diffusion imaging with compressed sensing: initial experience. Neurosurgery, 72(1), 165.
Li X. (2014) Diffusion-Weighted Imaging Analysis. In: Functional Magnetic Resonance Imaging Processing. Springer, Dordrecht. https://doi.org/10.1007/978-94-007-7302-8_5
Pierpaoli, C., Jezzard, P., Basser, P. J., Barnett, A., & Di Chiro, G. (1996). Diffusion tensor MR imaging of the human brain. Radiology, 201(3), 637-648.
Takahashi, E., Song, J. W., Folkerth, R. D., Grant, P. E., & Schmahmann, J. D. (2013). Detection of postmortem human cerebellar cortex and white matter pathways using high angular resolution diffusion tractography: a feasibility study. Neuroimage, 68, 105-111.
Tuch, D. S. (2004). Q‐ball imaging. Magnetic Resonance in Medicine: An Official Journal of the International Society for Magnetic Resonance in Medicine, 52(6), 1358-1372.
[i]Schilling, Kurt, Yurui Gao, Vaibhav Janve, Iwona Stepniewska, Benett A Landman, and Adam W Anderson. “Confirmation of a Gyral Bias in Diffusion MRI Fiber Tractography,” August 4, 2017. https://doi.org/10.1002/hbm.23936.
Comments