Inter-rater Variability in Presenting Radiological Data Using Brain Tumors
- Esha Choudhary
- Aug 16, 2022
- 6 min read
“Inter-rater reliability is the level of agreement between two or more individuals who measure or categorize the same objects or actions” (1). In other words, inter-rater reliability is the extent of agreement on a particular data collected among individuals.
On the other hand, inter-rater variability refers to the degree or extent to which there is disagreement or non-acceptability of a particular data among multiple observers or raters. If the inter-rater reliability has a greater measure the interpretation/ outcome is more accurate, whereas the higher the inter-rater variability less accurate will be the result.
Growth of Modern Medical Imaging
Earlier, if a doctor wanted to avoid investigative surgery they would have only one method of looking inside a patient: The X-ray machine, which was invented in 1895, and was the only way of looking inside the body for many decades. As X-rays can only show bones and certain large masses, small but aggressive cancer tumors would go unnoticed. This would lead to missing the cancer diagnosis until the tumor increased in size and turned fatal.
In the 1970s, researchers discovered a technique to use computers to combine several X-rays from different angles, called the CT scanner which allowed doctors to examine interior organs in 3-D with more clarity. The drawback is that it can reveal a mass but not what the tumor is doing, that is, if it is active and developing or whether it is non-cancerous. PET technology, which makes even the smallest tumor glow like a light in the dark, provided answers to these issues. It helped us comprehend the metabolic activity inside a tumor, which contributed to better diagnosis and prognosis of a patient's effects and survival. However, in the absence of anatomical landmarks, pinpointing a tumor might be difficult.
Following that, PET was paired with an MRI and obtained additional information which aids us in difficult circumstances such as when a tumor is located in the brain or liver. Diffusion MRI, perfusion MRI, and CT scans give more information regarding vascular proliferation and cell density and are used to differentiate between calcification and bleed. All of these advancements in the field are hugely dependent and have been possible because of human intervention and innovation. (2)
With cancer cases on the rise, diagnosing it in its early stages with standard methods has grown increasingly challenging. Diagnostic mistakes such as overlooked, delayed, or incorrect instances are common in traditional procedures. Meanwhile, comprehending the complexities of cancer—early diagnosis, accuracy, tumor development, growth patterns, aggressiveness, and defining the margins at different stages—has hindered future study. With the evolution of technology, radiology is now capable of predicting tumor mutation, treatment response, cancer prediction, and survival, hence enhancing medical care and patient survival.
For prolonged patient survival, it is essential to know the accurate location, size, and classification of tumor subtype, to plan optimum treatment/surgery.
Tumor Segmentation
Tumor segmentation allows us to do this by ‘marking’ or ‘isolating’ and classifying tumor and healthy tissues. While cancer research has advanced because of regular human intervention, it is still greatly dependent on human interpretation. For example, two radiologists might have different experiences and might present different interpretations and observations on the same case. This variability in the interpretations and the outcome remain a big hassle (7,8).
Consequently, segmentation outcomes often depend on the attributes of a rater. It is recommended to assign multiple raters so that they create independent readings that can provide us with a more accurate measurement of probable changes of a certain segmentation among the raters. In practice, the segmentation technique should be strong and have a high rater agreement.
Brain tumors could be cancerous or noncancerous growth/mass of abnormal cells in the brain. In one case as per the BraTS protocol, radiologists look at multiple MRI sequences.
The imaging protocol consists of -
T1 weighted scan in which anatomy can be observed
T2 weighted sequence, which is linked with edema and can show a measure of cellularity.
T1 contrast sequence, helps us differentiate among the regions with a disrupted blood-brain barrier from areas of the non-disrupted blood-brain barrier
Fluid Attenuation Inversion Recovery (FLAIR), helps us identify edemas.(3)

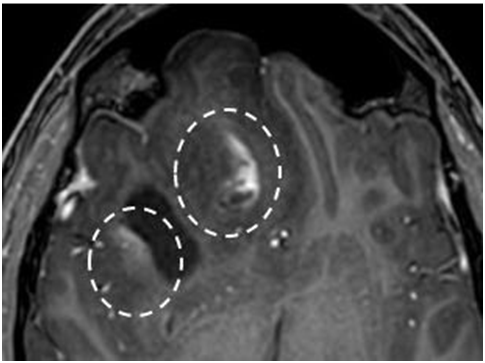
Image 1 - Depiction of a complex tumor lesion in the right frontal lobe. The key tumor parts (edema, enhancing tumor, necrotic core, etc) are hard to distinguish, as they have ill-defined tumor borders (right image- arrows) also it has vague contrast enhancement (left image- dashed circles) (3)
Gliomas stem in the glial cells and are the most common brain tumor. Based on the pathological assessment of the tumor, they can be divided into glioblastoma (GBM/HGG) and low-grade glioma (LGG). Glioblastoma is one of the most fatal brain tumors. On MRI, the diagnosis and evaluation can be quite subjective and it does not consider many quantitative features which is a major issue. (6)
Segmentation of a brain tumor consists of many steps, lesions or other tissues of interest (areas affected by tumor) have to be diagnosed, delineated, followed by the identification of different tissue types. In terms of brain tumor, the enhancing region edema and the necrotic core has to be separated from the healthy tissues. These are difficult to distinguish as the tumors are very heterogeneous. Each has a different size, shape, and location, tumor boundaries are irregular, ill-defined, and have faint contrast enhancement and discontinuities. On top of that, there are different imaging techniques and protocols which makes the standardization and data quality complicated.
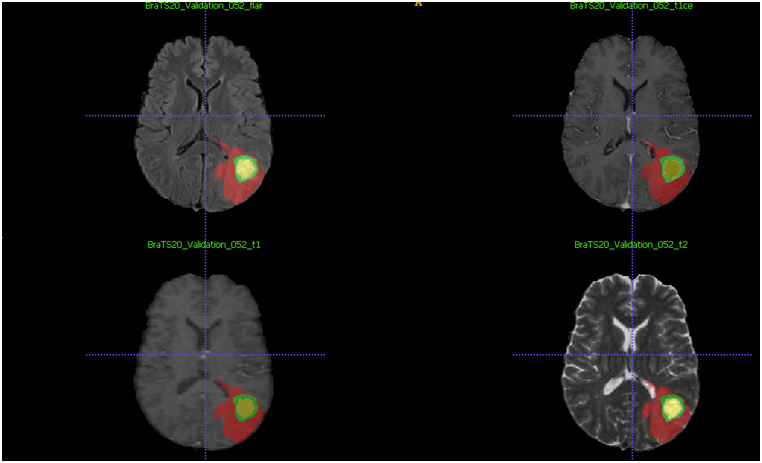
Image 2 - Depiction of a segmentation (automatic and minor corrections with manual segmentation) of a gliomas brain tumor and its different regions (red-edema, green–enhancing tumor, yellow–necrotic core) in different weighted sequences flair, t1c, t1, t2( in the same order) (10)
Diagnostic Accuracy in Radiology
An accurate diagnosis and segmentation are very important to assess response to therapy. Because each rater has a different interpretation and many ways for segmentation, it is crucial to know the range and measure of inter-rater variation. The measure of inter-rater variability can be evaluated on some basic principles which are repeatability and reproducibility. They are quantified using appropriate tests.
In most cases, segmentation by novices is compared to experts in the field. They receive training on how to operate the program before using it on MRI scans. Raters are informed of all criteria and are blind to the histological diagnoses and clinical follow-ups of the patients. However, no segmentation rules are imposed. Additionally, the sets are randomized
and the co-raters are blinded to their segmentations.
Ideally, inter-rater variability should be small. Some of the methods to measure inter-rater reliability and variability are:
The Kappa Coefficient is used to evaluate inter-rater consistency. It also accounts for agreement due to chance alone.
The intraclass correlation coefficient (ICC) is used when the measures are quantitative. (4)
Evaluation of the agreement and variability is done through various calculations and methods: majority voting over multiple raters is a good method to get consensus segmentation is better than a single rater’s segmentation. Overlap agreement is also observed between each rater’s segmentation and the majority vote for experts and novices are also integrated (4,5).
Inter-rater variability can be reduced and reliability can be improved by training data raters, providing them with a guide and standard for recording their observations, monitoring the quality of the segmentation and data interpretation over time to remove human bias, and offering a chance to discuss difficult issues or problems. Reliability can also be improved by increasing the number of raters, using good diagnostic instruments, and improving them.
The problem with the integration of outcomes from experts and novices is that it may incorrectly overlook the performance of individual raters and lead to oversimplification. It is also difficult to obtain an interpretation of segmentations from multiple experts.
AI powered Radiology is a potent amalgam
With the advent and evolution of Artificial intelligence in the medical field, the future looks promising. AI has radically changed the field of radiology, with the development of the Computer-Aided Detection (CAD) strategy. These approaches give us extremely accurate diagnosis as the detection and classifying capabilities of CAD systems are expert level.(3,5)
Manual segmentation of tumors is a very painstaking task prone to inter-rater bias, minimal accuracy, time-consuming, and inconsistent reproducibility. On the other hand, AI-based techniques give us a more standardized method, increased quality, reproducibility, and efficiency. Problems such as inter-observer variability, defining and evaluation of tumor heterogeneity, and classification may be solved. AI methods are either semi-automated or fully automated, they use characteristics involving symmetry, intensity gradients, etc to classify different regions more effectively. There are also novel deep-learning frameworks present for the segmentation of brain tumors and their sub-regions from MRI scans which provide highly improved survival predictions based on the segmentation.
Tumor progression and response to therapy must be correctly and accurately determined with the help of technologies and techniques that show the smallest possible measurement variability as it is crucial for better treatment and survival of the patient. The advantages of using these sophisticated, noninvasive, effective technologies are evident: more accurate and time-saving detection and diagnosis of the disease has translated into refined patient care
The constant pursuit for improvement in healthcare will undoubtedly give rise to many innovative, unimaginable new diagnoses and treatment methods.
References
Wennberg, A. Karlsen, Stalfors, Bratt & Bugten, S. L. J. M. V. (2019, January 7). Providing quality data in health care. Https://Bmcmedresmethodol.Biomedcentral.Com/Articles/10.1186/S12874-018-0651-2. https://bmcmedresmethodol.biomedcentral.com/articles/10.1186/s12874-018-0651-2
The Evolution of Medical Imaging for Cancer Care. (2020, October 20). IAEA. https://www.iaea.org/newscenter/multimedia/videos/the-evolution-of-medical-imaging-for-cancer-care
Challenges in brain tumor segmentation. (2020, August 19). Https://Healthcare-in-Europe.Com/En/News/Challenges-in-Brain-Tumour-Segmentation.Html. https://healthcare-in-europe.com/en/news/challenges-in-brain-tumour-segmentation.htm
Inter-rater agreement in glioma segmentations on longitudinal MRI. (2019, February 22). PubMed Central. https://www.ncbi.nlm.nih.gov/pmc/articles/PMC6396436/
Is this good enough? On expert perception of brain tumor segmentation quality. (2022, April 4). SPIE.DIGITAL LIBRARY. https://www.google.com/url?q=https://www.spiedigitallibrary.org/profile/Raymond.Huang-4355370&sa=D&source=docs&ust=1658221102107198&usg=AOvVaw2_wjN52hPGptJfdGGezPxK
Interobserver variability in the radiological assessment of response to chemotherapy in glioma. (2003, April). ResearcgGate. https://www.researchgate.net/publication/10863506_Interobserver_variability_in_the_radiological_assessment_of_response_to_chemotherapy_in_glioma
Agreement and Observer Variability. (2018, February 7). ScienceDirect. https://www.sciencedirect.com/science/article/pii/S2211568418300172
Interobserver agreement issues in radiology. (2020, October). Https://Www.Sciencedirect.Com/Science/Article/Pii/S2211568420302175. https://www.sciencedirect.com/science/article/pii/S2211568420302175
Image 1- Challenges in brain tumor segmentation. (2020, August 19). Https://Healthcare-in-Europe.Com/En/News/Challenges-in-Brain-Tumour-Segmentation.Html. https://healthcare-in-europe.com/en/news/challenges-in-brain-tumour-segmentation.html
Image 2 - Images taken from the BraTS dataset and are directly taken from the segmentation
Comentários