The Brain: A Multilayered Object
- Varsha Raghuram
- Sep 27, 2022
- 5 min read
Author: Varsha Raghuram
Editor: Dr. Rimjhim Agrawal
Multidimensional analysis is a fancy term. It seems like jargon restricted to the labs of computer scientists, but it can be as simple as a Rubik’s cube. Each side of the cube has a different colour, and every square carries one “unit” of that colour, which is essential to solving that side of the cube. So the Rubik’s cube can be studied and understood from three dimensions: The cube as a whole, when solved for all 6 sides, each of the 6 sides of the cube, when solved for their colour, and the individual squares of colour.
This can be called ‘multidimensional analysis’ - the separate understanding of the data points that make up the Rubik’s cube, those data points being organised in a meaningful hierarchy (Whole cube -> each side -> individual squares). It allows you to view data from various points, helping you spot a trend or an exception in the data.
But how is any of this related to the brain?
Network theory- structural and functional networks
The network theory in mathematics has proved to be a promising approach to studying the structural and functional organisation of brain units. So far, Diffusion Tensor Imaging (DTI) is being used with histological data to evaluate structural connection patterns by measuring the physical links (including white matter) between neurons and regions. DTI uses diffusion of water molecules and the result of their interaction with tissues to measure these with high accuracy, allowing reconstruction of nerve fibres and three-dimensional mapping of the human brain with incredible resolution. (fig. 1)
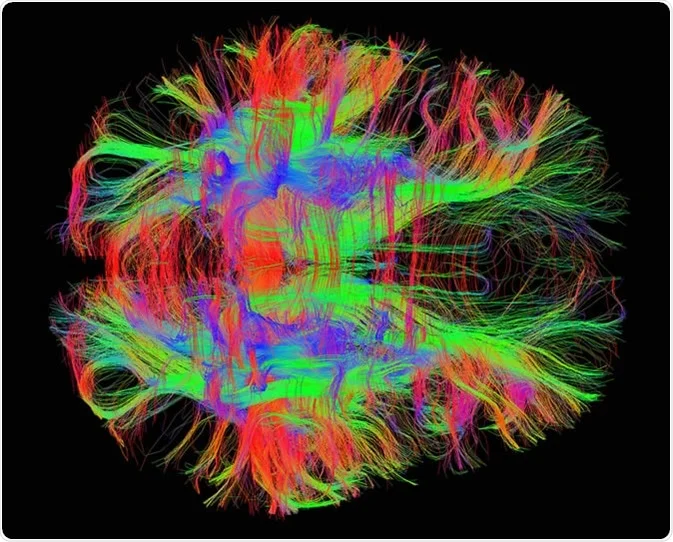
Image credit: Zeynep Saygin, mcgovern.mit.edu
However, the functional networks are studied using functional MRI, measuring physical signals like blood oxygen levels from different regions, then comparing pairwise signals by some similarity measure. (fig. 2)
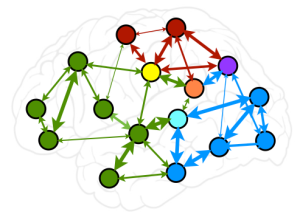
Image credit: David Papo, wordpress.com
Network modelling approaches successfully unveiled many interesting features, including but not limited to small-worldness - where the underlying topology is highly locally clustered and the presence of long-range connections dramatically reduces the distance between units; and modular and rich-club organisation - where the underlying topology can be described as a network of modules with highly-connected units that tend to connect to each other more often than random chance would predict. The success of network mapping has also highlighted the need for new methods of probing the structure and the function of the brain on multiple levels.
Still, the lack of an appropriate mathematical framework for the representation and analysis of multivariate connectivity data forced many studies to neglect, disregard, or approximate available information to cope with the high amount of underlying complexity. In simple terms, there are connections in the brain that overlap, and two regions of the brain - analogous to nodes in artificial neural networks (ANNs) - could have more than one connection - analogous to the edges in ANNs. Researchers often had to ignore or approximate other connections to derive standalone meaning from just one set of nodes and edges.
Yet, we may no longer need to disregard data if we take a new angle. Remember the concept of multidimensional analysis? That comes into play with an interesting type of network, called the multilayer network. The name is descriptive enough - these networks consist of multiple layers of connections and subsystems. A subtype of the multilayer network, called a multidimensional network, specifies that there are as many dimensions (think about the Rubik’s cube again - the dimensions being the cube, the sides of the cube, and the individual squares) as there are layers (connection of the cube to each side, each side to the coloured squares, and the squares to the whole cube) in the network.
Now we can consider the brain as a multidimensional network, and thus apply multidimensional analysis in studying it. Researchers at the Blue Brain Project have performed pioneering work in studying the brain as a multidimensional network, and they say the potential is huge. In 2017, they generated a computer model where the brain was made up of multidimensional geometrical structures and spaces. It was reported that there were billions of these objects even in a tiny speck of the brain, easily reaching seven dimensions. In some networks, they even found structures with as many as eleven dimensions (fig. 3).
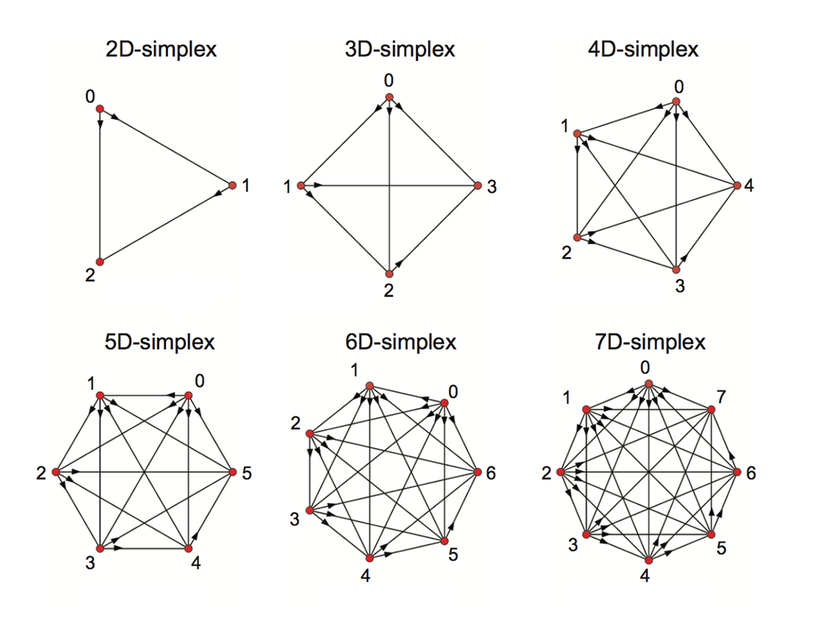
Image credit: The Blue Brain Project, retrieved from cosmosmagazine.com
These multidimensional structures are formed through a phenomenon known as neural cliquing. Neural cliques occur in the hippocampus, the area of memory storage, and - just as multidimensional analysis requires! - are organised in a categorical, hierarchical manner. According to the theoretical memory model called the ‘Gripon-Berrou Neural Network’ or ‘Cliques Neural Network’, the encoding of memories happens in a constant amount of time, regardless of the size of the memory. This occurs by just creating synapses between similar neurons in the hippocampus, and these similar neurons come together to form a clique. The neurons all connect via synapse, and form a new object, which creates the multidimensional structure observed by the researchers. The more neurons there are in a clique, the higher the so-called dimension of the object (fig. 4).
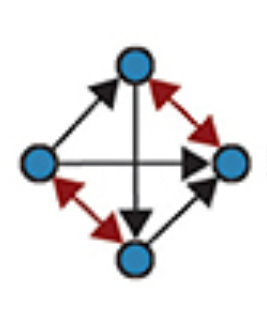
Image credit: Reimann et al., frontiersin.org
But, as is often the case in mathematics, all the ideas of seven and eight dimensions are just theoretical. In actual space, these neural clique-created structures cannot exist in more than three dimensions. So why even bother applying multidimensional analysis? We do this because we don’t yet have the clarity of imaging and advancement in science to study the brain in just three dimensions, and multidimensional networks are by far the closest we have gotten to an approximate understanding of the brain.
There are many questions that the applicability of multidimensional networks to neuroscience raises. Considering the massive growth of ANNs and the way we are using theory behind them to study the brain itself, it’s worth considering the concept of technological singularity with the brain. The singularity is a hypothetical point in time at which technological growth will become uncontrollable and irreversible, going on to ‘run away’ with its own ability and create a superintelligence that far surpasses human intelligence qualitatively. But just how complex is this singularity? Is it anywhere near as complex as the concept of consciousness; or to take a simpler approach, as complex as the structure of the universe? Those questions lead you to ask if there is any similarity in the structure of the universe and the brain, which brings us back to the concept of networks - because the universe is simply one huge network of interconnected factors.
We may never know, or we might know tomorrow. Either way, multidimensional analysis is a field blooming with potential and exploring it could be the greatest blessing that computational neuroscience has seen so far.
Citations
De Domenico, M. (2017, May 1). Multilayer modeling and analysis of Human Brain Networks. GigaScience. Retrieved from https://ncbi.nlm.nih.gov/pmc/articles/PMC5437946/
Vaiana, M., & Muldoon, S. F. (2018, January 3). Multilayer Brain Networks - Journal of Nonlinear Science. SpringerLink. Retrieved from https://link.springer.com/article/10.1007/s00332-017-9436-8
Beall, A. (2017, June 12). The multi-dimensional Universe hiding inside your head. WIRED UK. Retrieved from https://www.wired.co.uk/article/neurons-multi-dimensional-network-brains
Gosztolai, A., & Arnaudon, A. (2021, July 27). Unfolding the multiscale structure of networks with Dynamical Ollivier-Ricci curvature. Nature News. Retrieved from https://www.nature.com/articles/s41467-021-24884-1
Tsien, J.Z. (2015, October 22). The power of two: Neural cliques provide a prewired framework for how neurons connect. Neuroscience News. Retrieved from https://neurosciencenews.com/connectivity-theory-neuroscience-2917/
Giusti, C., Pastalkova, E., Curto, C., & Istkov, V. (2015, October 20). Clique topology reveals intrinsic geometric structure in neural correlations. Proceedings of the National Academy of Sciences of the United States of America. Retrieved from https://pubmed.ncbi.nlm.nih.gov/26487684/
Mandke, K., Meier, J., Brookes, M. J., O'Dea, R. D., Mieghem, P. V., Stam, C. J., Hillebrand, A., & Tewarie, P. (2017, November 11). Comparing multilayer brain networks between groups: Introducing graph metrics and recommendations. NeuroImage. Retrieved from https://www.sciencedirect.com/science/article/abs/pii/S1053811917309230?via%3Dihub
Kivelä, M., Arenas, A., Barthelemy, M., Gleeson, J. P., Moreno, Y., & Porter, M. A. (2014, July 14). Multilayer Networks. OUP Academic. Retrieved from https://academic.oup.com/comnet/article/2/3/203/2841130
Comments